At SHIFT, our approach is to apply equal parts art and science to build integrated programs that help brands connect with the people that matter most. But what does the 'science' part of communications entail? What does it look like in action?First and foremost, it means to be data-driven in our planning and execution; to make informed decisions based on data and research. In this series, we examine how to become a more data-driven communications professional.
Refining our hypothesis
In the previous post we proved this hypothesis false: Trustworthy content is shared more on social media.If this was our big idea for our PR pitch, we're probably feeling a little uncomfortable right now.The next logical question we should consider is whether this false hypothesis is universally false. Is it always the case that trustworthy content is not shared more on social media? Is there some refinement we could make to our data?
Changing perspective
One of the most useful tactics to take is to change our perspective. The last time we looked at this data, we explored it as a linear series, a bar graph. What if we looked at our data more granularly? Suppose we plotted trust and sharing as a two-dimensional plot?

What we see instead of a neat line graph is something else entirely: three clusters of social media sharing. While trust is distributed relatively evenly, social media sharing has a highly irregular pattern of data.What would we expect from a list of URLs in terms of social media sharing? We would typically expect a power law distribution, also known as an 80/20, where the majority of sharing occurs in a few URLs.The original chart obscured this highly unusual distribution. What might we conclude about our hypothesis? Based on this data, the most likely conclusion is that the data itself is flawed. The hypothesis, rather than being definitively false, is still unproven because our source data appears to be bad.
Refining our data
Let’s say we fixed our data. We found an anomaly and cleaned the data to ensure the anomaly was no longer present. What might we determine now?

What we see in the cleaned data above is a different pattern; we see a completely normal distribution. The majority of links have medium to high trust, and the majority of links have medium to high sharing.

Now that we know our data is clean, what conclusions might we draw from this? Does this prove or disprove our hypothesis? Statistically, if we run a Pearson regression analysis, we see:
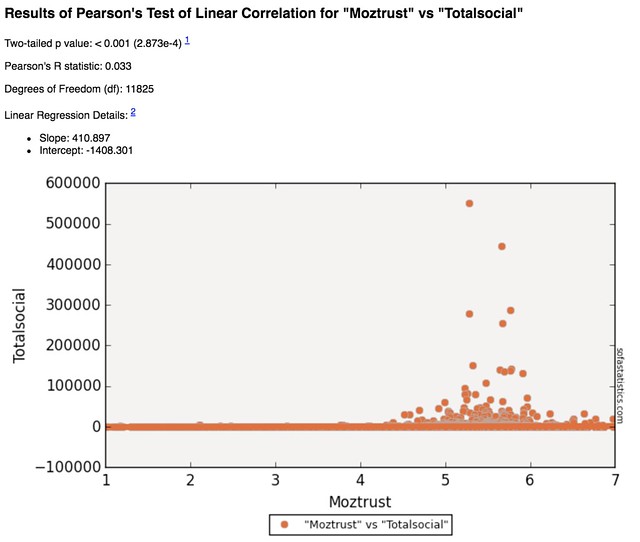
Unfortunately, the statistical outcome is the same: social sharing and trust still have no relationship to each other.
The verdict stands
Our hypothesis, Trustworthy content is shared more on social media, is still false, even with a refined, cleaned data set. Could we refine it further, to test for conditions under which it might be true? Yes. We could restrict URLs to just specific industry sectors or business types to see if those variables make a difference. To do so, we’d need to refine our hypothesis to clarify we were testing just that assumption.This is the moment that sets apart regular PR from data-driven PR. In regular, traditional PR, we might have a pitching point given to us by our stakeholders or that we invented. At this point in the process, we have essentially invalidated the pitching point. What a quandary! An ethical PR professional would at this point need to go back to the stakeholders and say that our idea was invalid.Where the data-driven PR professional shines is asking the questions:How else might we refine this?What else does our data tell us?Is there a different but equally interesting story here?If we cared about social sharing more, or we cared about trust more, we might want to see what other variables influence the target of our choice. Perhaps sharing and trust don’t have a relationship, but trust and something else might. Using a tool like IBM Watson Analytics, we could do more advanced statistical analysis like linear analysis of variance to determine what these other variables could be.

Above, we see that trust is driven by inbound links, linking domains, and individual page authority. Based on this information, we might alter our hypothesis to say:Trustworthy content is linked to more on the web.Based on the analysis above, we would conclude this hypothesis is true. While we don't have our original hypothesis, our original pitching point, we do have something verifiably true to work with.
Next: pitching
We’ve now established a true hypothesis, though it wasn’t the hypothesis we began with. What do we do with it? How do we transform our data, our analysis, and our insight into pitch worthy material? In the next post, we’ll explore how to craft a data-driven pitch.
What’s a Rich Text element?
The rich text element allows you to create and format headings, paragraphs, blockquotes, images, and video all in one place instead of having to add and format them individually. Just double-click and easily create content.
The rich text element allows you to create and format headings, paragraphs, blockquotes, images, and video all in one place instead of having to add and format them individually. Just double-click and easily create content.
Static and dynamic content editing
A rich text element can be used with static or dynamic content. For static content, just drop it into any page and begin editing. For dynamic content, add a rich text field to any collection and then connect a rich text element to that field in the settings panel. Voila!
How to customize formatting for each rich text
Headings, paragraphs, blockquotes, figures, images, and figure captions can all be styled after a class is added to the rich text element using the "When inside of" nested selector system.